
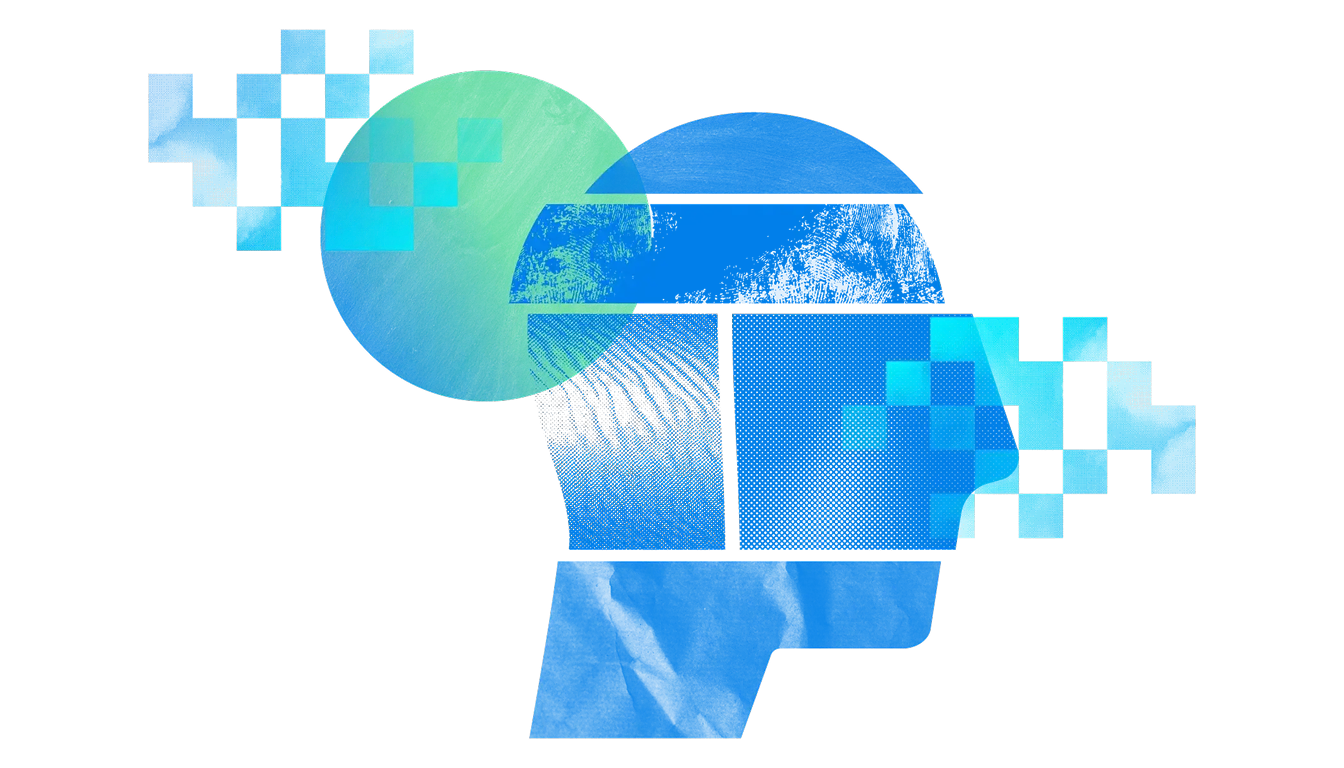

Responsible AI
The Democratic Dilemma: AI Alignment and Social Choice Theory
Apr 30, 2025
5 min read
Introduction
As artificial intelligence systems grow more capable and integrated into our daily lives, the question of how to make them behave in accordance with human values becomes increasingly critical. This challenge, known as AI alignment, sits at the intersection of computer science, ethics, and social policy. But a fundamental question remains insufficiently addressed: whose values should these systems reflect?
This question has become even more pressing with the rise of Large Language Models (LLMs) like GPT-4, Claude, and Llama, which power many of today's most widely used AI applications. These systems have been trained using a technique called Reinforcement Learning from Human Feedback (RLHF), which has proven remarkably effective at making AI outputs safer, more accurate, and more helpful. Yet this approach brings its own challenges when we consider how to implement it in a way that respects democratic norms.
The RLHF Revolution
Reinforcement Learning from Human Feedback has transformed AI development. In this approach, human evaluators provide feedback on AI outputs, effectively teaching the system which responses are desirable and which should be avoided. The AI learns to maximize "rewards" based on this human feedback, gradually aligning its behavior with human preferences.

This method has been crucial for mitigating potentially harmful outputs from powerful AI systems, helping them avoid generating biased, toxic, or misleading content. All major deployed language models now use some version of RLHF for fine-tuning their outputs.
But this raises a crucial question: who are these human evaluators, and how representative are their values?
The Social Choice Problem
This is where social choice theory—a field that studies how individual preferences can be aggregated into collective decisions—becomes relevant. In a democratic society, we might want AI systems that reflect broad societal values rather than the preferences of a small group of evaluators or the companies that employ them.
Research by Abhilash Mishra at Equitech Futures addresses this challenge head-on, drawing on established impossibility results in social choice theory. Mishra's work shows that under broad assumptions, there is no unique, universally satisfactory way to democratically align AI systems using RLHF. This is not merely a theoretical concern but a practical challenge for anyone attempting to build AI systems that respect diverse human values.
Arrow's Impossibility Theorem: The Voting Paradox
To understand why democratic AI alignment is so challenging, we need to examine Kenneth Arrow's groundbreaking work in social choice theory. In 1951, Arrow—who would later win a Nobel Prize for his contributions to economic theory—proved a startling result: no voting system can simultaneously satisfy a set of seemingly reasonable fairness criteria when there are three or more options to choose from.
The criteria Arrow considered were:
- Universality: The voting system must produce a valid ranking for any possible combination of individual preferences
- Non-dictatorship: No single individual's preferences should determine the outcome regardless of others' preferences
- Independence of irrelevant alternatives: If an option is removed, the relative rankings of the remaining options should not change
- Pareto efficiency: If everyone prefers option A to option B, then A should be ranked above B in the final outcome
Arrow mathematically proved that no voting system can satisfy all these criteria simultaneously. This means that any democratic decision-making process must inevitably violate at least one principle we might consider essential to fairness.
In the context of AI alignment, Arrow's theorem has profound implications. When we attempt to aggregate the preferences of multiple human evaluators to determine how an AI should behave, we inevitably run into this impossibility result. Should we prioritize the majority view? Protect minority perspectives? Favor experts? Each choice involves trade-offs that cannot be resolved through any perfect voting mechanism.
Sen's Liberal Paradox: Individual Rights vs. Collective Welfare
While Arrow's theorem focuses on the challenges of preference aggregation, Amartya Sen's Liberal Paradox highlights another fundamental tension in social choice: the conflict between individual liberties and collective welfare.
Sen, another Nobel laureate, demonstrated in 1970 that it is impossible to have a social decision function that simultaneously satisfies:
- Minimal liberalism: Each person should have control over at least some personal decisions
- Pareto principle: If everyone prefers option A to option B, society should prefer A to B
- Unrestricted domain: All preference profiles are admissible
Sen's paradox reveals that even minimal protections for individual rights can come into conflict with unanimous collective preferences. This creates situations where respecting everyone's personal freedoms leads to outcomes that everyone agrees are worse overall.
Applied to AI alignment, Sen's paradox illuminates why we cannot create systems that both respect individual autonomy and maximize collective welfare. Should an AI respect a user's potentially harmful preferences if they only affect that user? Should it override personal choices if they contribute to collectively negative outcomes? These questions have no perfect answers within Sen's framework.
To explore this further, consider the following thought experiment through the framework outlined above:

The Reinforcer's Dilemma: Impossibility in Practice
To understand this challenge more concretely, consider a scenario where AI evaluators (reinforcers) have different preferences regarding how a system should respond to potentially controversial topics.
Imagine Reinforcer A prefers that the AI remain neutral on divisive issues, while Reinforcer B would rather the AI clearly state a position. If we try to determine the "best" output through a voting mechanism, we quickly encounter paradoxes and contradictions.
For example, if we have three possible outputs:
- A response that takes position A
- A response that takes position B (opposite to A)
- A neutral response that avoids taking either position
Different reinforcers might rank these options differently based on their own values and priorities. One might prefer neutrality above all (ranking option 3 highest), while another might prioritize clear position-taking (ranking options 1 or 2 highest).
When we try to aggregate these preferences into a single "best" output, we run into the exact challenges that social choice theory has identified: no matter what aggregation method we use, we will violate some principle of fairness or democratic decision-making.
Beyond Universal Alignment
This research has profound implications for AI governance and development. If we cannot achieve a universally aligned AI through democratic processes, what alternatives exist?
Mishra suggests that instead of pursuing a single "aligned AGI" (Artificial General Intelligence) that would satisfy everyone, the field might be better served by developing multiple narrowly aligned AI systems, each designed to serve the needs and reflect the values of specific user communities.
This approach embraces pluralism rather than trying to resolve fundamental value disagreements. It suggests a future with diverse AI systems reflecting different value systems, rather than a single universal AI that attempts to satisfy everyone but inevitably disappoints many.
Conclusion
As AI becomes more integrated into our social fabric, questions of alignment will only grow more important. Mishra's work highlights that these are not merely technical challenges but deeply social ones that engage with fundamental questions about how we make collective decisions in diverse societies.
The impossibility results from social choice theory don't mean we should abandon efforts to align AI with human values. Rather, they suggest we need more sophisticated approaches that acknowledge inherent tensions and trade-offs. They also invite humility about what can be achieved through purely technical means, reminding us that some of the most important questions in AI development are ultimately political and ethical in nature.
As we navigate this complex landscape, researchers, policymakers, and citizens all have important roles to play in determining how AI systems will reflect and shape our collective values. The conversation about AI alignment is, at its core, a conversation about who we are and what kind of society we want to build.
Further Reading
For those interested in exploring these topics further, Mishra's original paper "AI Alignment and Social Choice: Fundamental Limitations and Policy Implications" provides a more technical treatment of these ideas, drawing connections between established results in social choice theory and the emerging challenges of AI alignment.
More recent work, including "Social Choice Should Guide AI Alignment in Dealing with Diverse Human Feedback" by a team of researchers from a December 2023 workshop at Berkeley, continues to develop the connections between social choice theory and AI alignment, offering practical suggestions for moving this important work forward.