
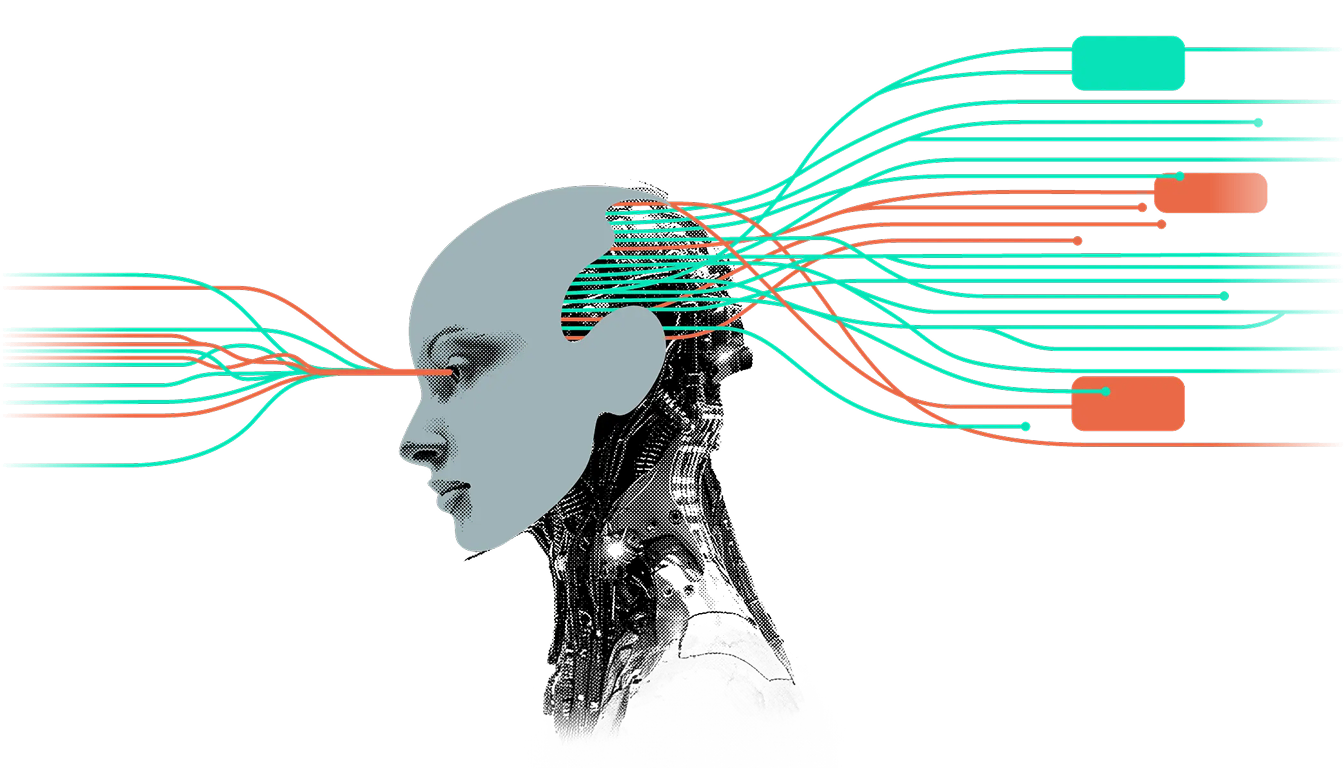
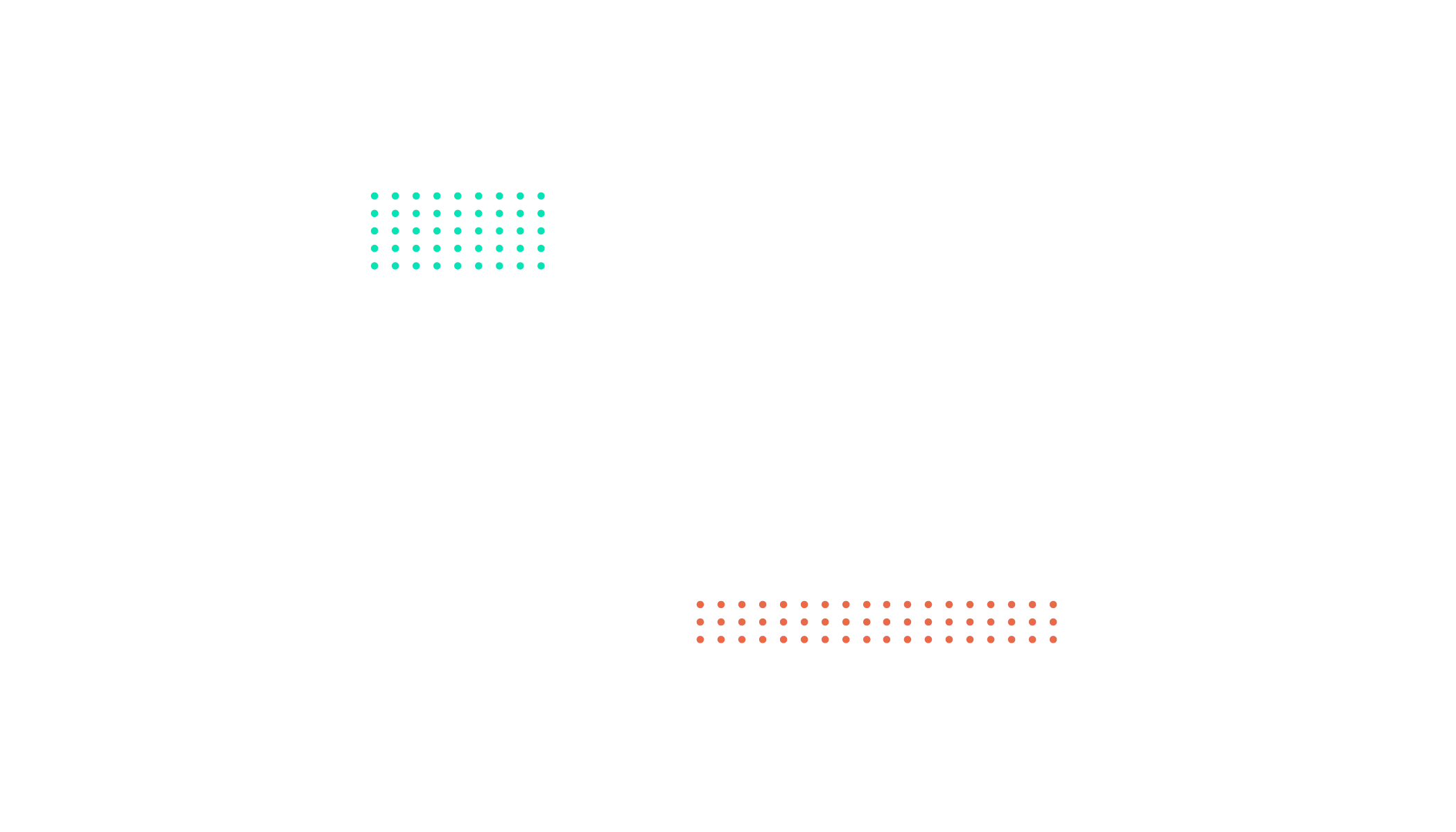
Responsible AI
Who Audits the Auditors? The Hidden Labels Problem in AI Fairness
Apr 30, 2025
5 min read
The Trust Crisis in AI
As artificial intelligence systems increasingly make decisions that affect human lives—from approving loans to diagnosing diseases to determining who gets released on bail—concerns about their fairness have prompted calls for greater oversight. A growing movement of researchers, journalists, and regulators has responded with algorithmic audits designed to detect bias and discrimination. These audits have revealed troubling disparities in how AI systems treat different demographic groups, spurring important conversations about the social impacts of technology.
But what if the very process designed to check for bias has a fundamental flaw?
In groundbreaking research conducted in India, scientists at Equitech Futures have uncovered a critical weakness in how we evaluate AI fairness: the quality of the "ground truth" data used to judge these systems in the first place. Their findings suggest that human errors in data labeling can create the illusion of algorithmic bias where none exists—or potentially mask real bias that should be addressed.
The Unexpected Discovery
When researchers Abhilash Mishra and Yash Gorana set out to evaluate the performance of commercial automated speech recognition (ASR) systems in India, they expected to find disparities similar to those documented in the United States, where these systems have been shown to perform worse for Black English speakers compared to white English speakers.
India provided an ideal testing ground with its rich diversity of languages, accents, and socioeconomic backgrounds. The team collected speech samples from over 100 high school and college students from both rural and urban areas, all of whom spoke English as a second language.
Following standard practice, they hired professional transcribers to create "ground truth" transcriptions of what was actually said in each recording. These human-generated transcripts would serve as the benchmark against which the accuracy of automated systems from Amazon, Google, and Microsoft would be measured.
The initial results were concerning. The AI systems appeared to perform significantly worse for rural speakers compared to their urban counterparts—a disparity that could have serious implications as voice-based technologies become increasingly common in educational and healthcare applications.
But as the researchers dug deeper, they noticed something unexpected.
The Three Iterations
Suspecting potential issues with the quality of the human transcriptions, the team implemented an iterative review process:
Iteration 1: The original transcriptions provided by freelance transcribers with backgrounds similar to data annotators typically employed by AI companies in India.
Iteration 2: A review focused on correcting instances where transcribers had "improved" the grammar of what was actually said, rather than transcribing the exact words.
Iteration 3: A meticulous check that captured all nuances of speech, including filler words (like "um" and "err") and incomplete utterances.
With each iteration, something remarkable happened: the apparent performance gap between how the ASR systems handled rural versus urban speakers began to shrink. By the final iteration, after the most rigorous data cleaning, the disparity had disappeared entirely.

The Labeling Paradox
What caused this surprising shift? The researchers discovered a critical pattern: human transcribers, who were predominantly urban English speakers themselves, unconsciously "corrected" grammatical errors and other speech patterns that were more common among rural speakers.
The ASR systems, in contrast, were faithfully transcribing exactly what was said—including grammatical errors and dialect variations. When these machine transcriptions were compared against the "corrected" human versions, the systems appeared to be performing poorly. In reality, they were often more accurate at capturing what was actually said than the human transcribers who were supposed to be providing the ground truth.
"This leads to a spurious reduction in ASR performance driven by human annotation errors," the researchers explain. The very process meant to check for bias was introducing its own systematic distortions.

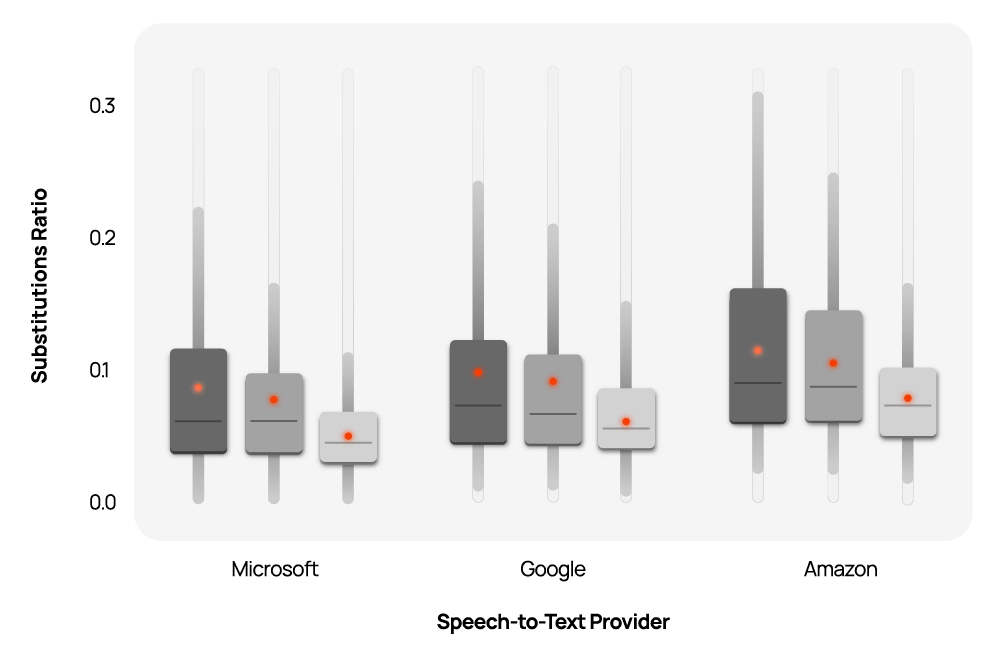
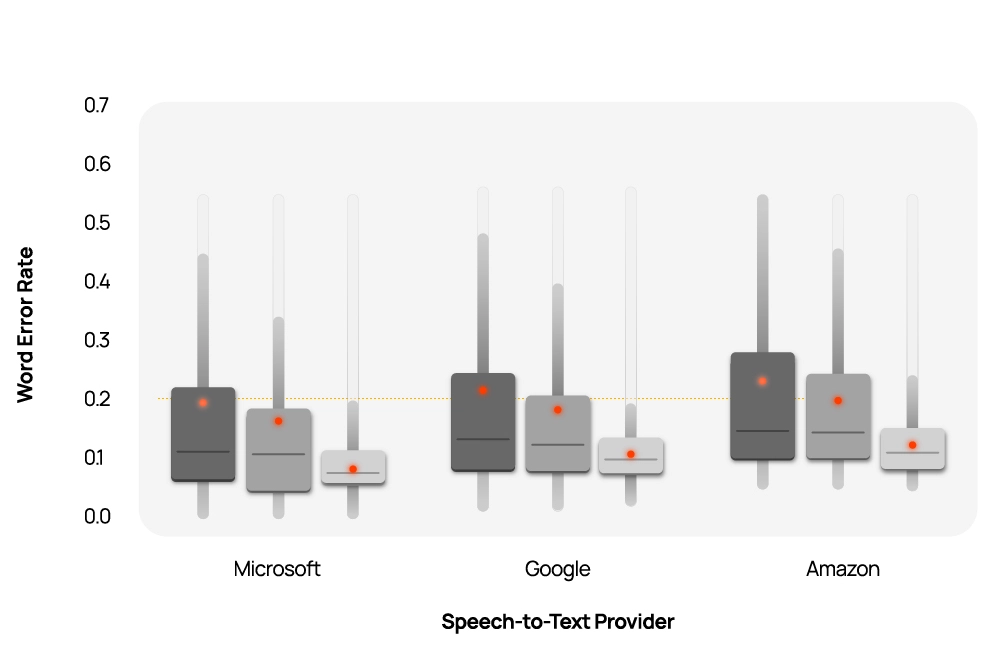
The Costly Truth
The implications extend far beyond speech recognition. Across AI applications—from computer vision to content moderation to healthcare diagnostics—human-labeled datasets serve as the benchmark for evaluating system performance and detecting bias. If these "ground truth" datasets themselves contain quality issues or implicit biases, they can severely distort our understanding of algorithmic fairness.
Properly cleaning and verifying these datasets is possible, but it comes at a steep cost. The researchers note that the thorough verification process they undertook was "rigorous, albeit expensive"—highlighting an uncomfortable economic reality. High-quality data labeling requires significant time and expertise, creating tensions between cost constraints and the integrity of fairness evaluations.
This raises difficult questions: How much should companies, researchers, and regulators invest in ensuring the quality of benchmark datasets? Who bears this cost? And what standards should the industry adopt to ensure that algorithmic audits are themselves trustworthy?
Beyond Technical Solutions
The labels problem in algorithmic auditing isn't merely technical—it's fundamentally a governance challenge. As the researchers argue, "There are no universal benchmarks for label quality. In the context of algorithmic auditing, label quality is both a normative and economic question."
Questions of whose interpretation counts as "ground truth" and what constitutes acceptable quality are inherently value-laden. In multilingual, multicultural societies like India, these questions become even more complex as technologies developed primarily in Western contexts encounter vastly different linguistic and cultural landscapes.
This research points to the need for consensus-driven, widely accepted protocols for data quality in algorithmic audits. Without such standards, well-intentioned efforts to ensure AI fairness may lead to misleading conclusions that either raise false alarms or provide false assurances.
Reimagining Algorithmic Auditing
Moving forward, the researchers suggest several approaches to strengthen algorithmic auditing:
- Transparent documentation of how benchmark datasets are created, including who the labelers are, what instructions they received, and what verification processes were used
- Multiple perspectives in the labeling process, especially ensuring representation from the communities most affected by the technology being evaluated
- Iterative verification similar to the three-stage process used in this study, with clear reporting of how results change across iterations
- Standardized protocols developed through multi-stakeholder collaboration between industry, academia, civil society, and regulators
As AI systems become more deeply embedded in critical social institutions, the stakes of getting fairness evaluations right only increase. This research reminds us that in the quest for algorithmic accountability, we must scrutinize not only the systems being assessed but also the assessment process itself.
After all, who audits the auditors?